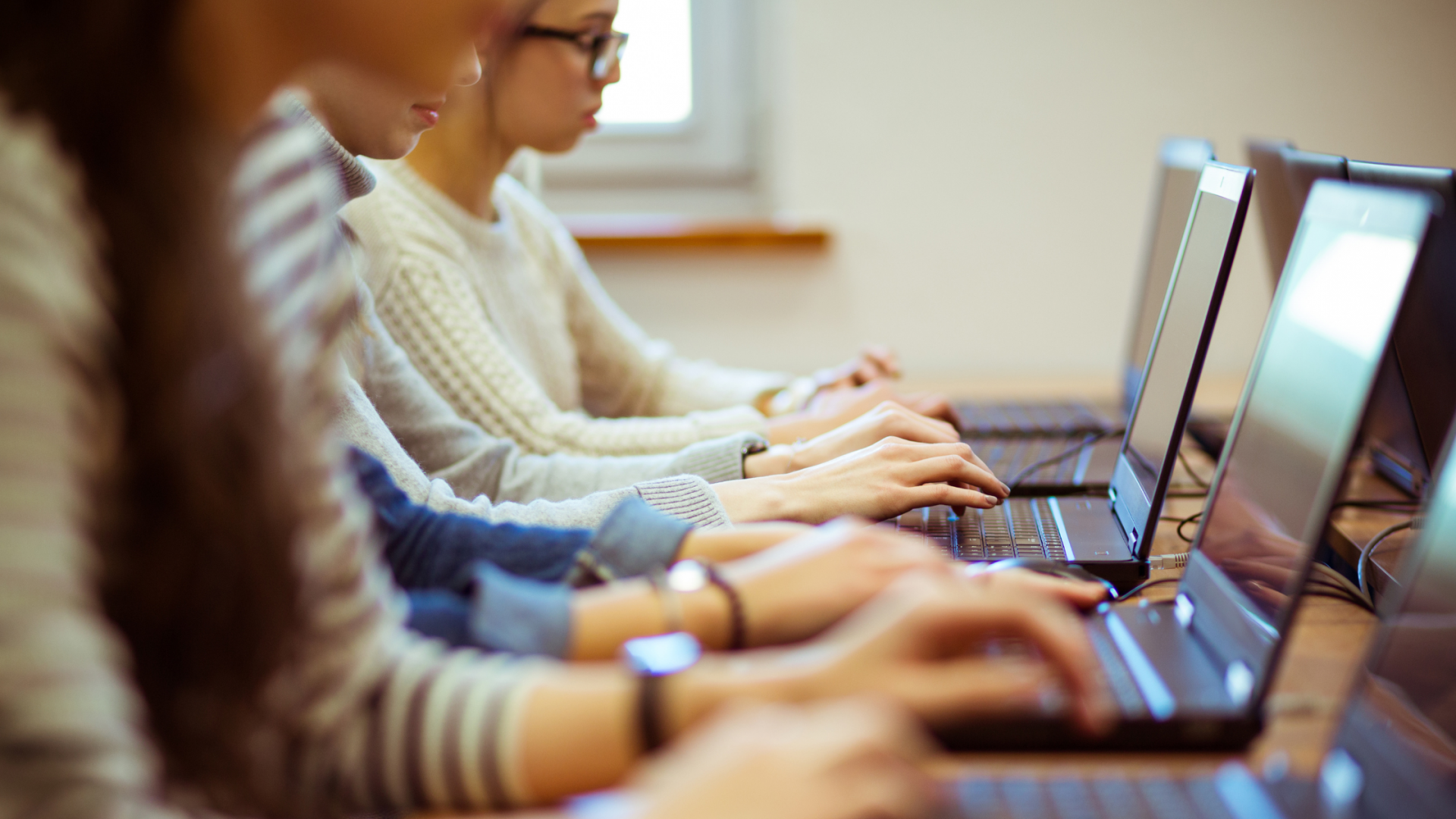
In the modern education landscape, the efficient management and analysis of student records are paramount to ensure personalized learning experiences and informed decision-making. With vast amounts of data generated by educational institutions, optimizing student record categorization becomes crucial for easy retrieval and analysis. Fortunately, the advancement in technology can aid us in categorizing these records. The introduction of digital tools, such as student record management system ensure structured data entry and retrieval. This article explores the importance of optimizing student record categorization and provides actionable strategies to streamline this process effectively.
Understanding the Significance of Student Record Categorization:
Accurate and efficient categorization of student records lays the foundation for seamless data management and analysis. Educational institutions can quickly access information about students by classifying data into relevant categories. Administrators, teachers, and other stakeholders may use this category to discover important information about students’ development, spot areas for improvement, and make well-informed choices to improve the learning environment.
Implementing a Structured Data Entry System:
To optimize student record categorization, institutions should establish a structured data entry system. This system should encompass standardized data fields and formats, ensuring consistency and uniformity across records. By providing clear guidelines for data entry, institutions can reduce errors, enhance data accuracy, and simplify the categorization process. Additionally, utilizing data validation techniques and automated checks can further streamline the entry process, preventing inconsistencies and improving the overall quality of student records.
Utilizing Hierarchical Categorization Frameworks:
One effective strategy for optimizing student record categorization is the use of hierarchical frameworks. These frameworks involve organizing data into multiple levels or tiers, allowing for categorization. For instance, student records can be categorized based on broad categories such as grade level, and then further subcategorized into specific subjects, attendance records, or disciplinary incidents. This hierarchical approach enables administrators to access relevant information quickly and analyze student data from various perspectives.
Implementing Metadata and Tagging Systems:
Incorporating metadata and tagging systems is another valuable technique to optimize student record categorization. By attaching metadata, such as keywords or labels, to student records, institutions can enhance searchability and retrieval. Additionally, tagging systems provide a flexible way to classify records based on specific criteria, such as academic achievements, medical conditions, or language proficiency. This enables educators and administrators to filter records based on specific attributes, simplifying data analysis and generating customized reports as needed.
Leveraging Data Analytics and Machine Learning:
To unlock deeper insights and optimize student record analysis, educational institutions can leverage data analytics and machine learning techniques. Advanced analytics tools can uncover patterns, trends, and correlations within student records, providing valuable information for personalized learning strategies. Machine learning algorithms can automate the categorization process by recognizing patterns and assigning records to appropriate categories automatically. This combination of analytics and machine learning empowers institutions to make data-driven decisions and offer tailored support to students.
Ensuring Data Privacy and Security:
As educational institutions optimize student record categorization, it is crucial to prioritize data privacy and security. Safeguard student records from any unauthorized access and breaches. Implement secure data storage, access restrictions, and encryption technologies. By maintaining a robust data protection framework, institutions can instill trust and confidence among students, parents, and staff members.
Optimizing student record categorization is a critical aspect of efficient data management and analysis in educational institutions. By implementing a structured data entry system, utilizing hierarchical frameworks, employing metadata and tagging systems, leveraging data analytics and machine learning, and prioritizing data privacy and security, institutions can streamline the categorization process. With easy retrieval and analysis of student records, educators and administrators can make informed decisions, personalize learning experiences, and improve overall student outcomes.